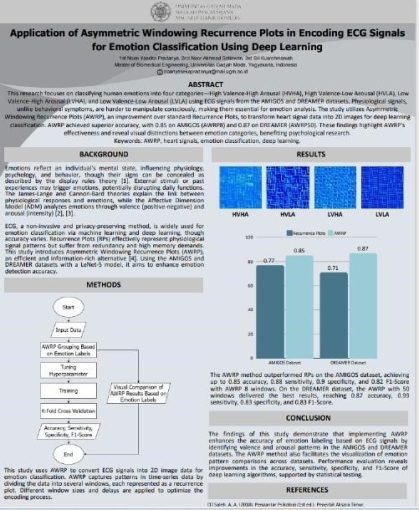
The relationship between physiological signals and human emotions has made Noan Yaseka interested in researching this, which was written in a thesis entitled Application of Asymmetric Windowing Recurrence Plots in Encoding Heart Signals for Emotion Classification Using Deep Learning
Guided by Ir. Noor Akhmad Setiawan, S.T., M.T., Ph.D., IPM from the Faculty of Engineering and Dra. Sri Kusrohmaniah, M.Sc., Ph.D., Psychologist from the Faculty of Psychology, Noan has successfully graduated with cum laude predicate from the Master of Biomedical Engineering Study Program, UGM Postgraduate School (SPs) and attended the graduation on January 31, 2025
Her thesis focuses on classifying human emotions into four categories: High Valence-High Arousal, High Valence-Low Arousal, Low Valence-High Arousal, and Low Valence-Low Arousal, by utilizing heart signal data from two public datasets, AMIGOS and DREAMER.
The primary objective of this research is to visually differentiate between valence and arousal levels while measuring the performance of deep learning algorithms in classifying human emotions. Emotions are typically manifested through three main symptoms: physiological, psychological, and behavioral. Physiological symptoms include changes in heart rate, blood pressure, and nervous system activity, while psychological symptoms involve mood and feeling changes. Behavioral symptoms encompass facial expressions and body movements.
However, since behavioral symptoms can be controlled or concealed, the analysis of physiological signals becomes increasingly important. These signals are challenging to manipulate consciously, making them a reliable source for emotion analysis. One of the methods employed in this research is Recurrence Plots (RPs). However, the redundancy patterns produced by this method can lead to misinterpretation, highlighting the need for further development.
To address this issue, the research implements Asymmetric Windowing Recurrence Plots (AWRP), an advanced method derived from RPs. This innovative approach transforms time series data of heart signals into two-dimensional (2D) images, enhancing the clarity and accuracy of emotion classification. The results of the study indicate that AWRP significantly outperforms traditional RPs, achieving the highest accuracy of 0.85 on the AMIGOS dataset using AWRP8, and 0.87 on the DREAMER dataset using AWRP50.
Moreover, the research reveals distinct visual differences between the four emotion categories, providing valuable insights into the emotional states of individuals. These findings have significant implications for the field of psychology, as they enable more accurate labeling of emotional types based on heart signals. This advancement aligns with the Sustainable Development Goals (SDGs) by promoting quality education and fostering innovation in biomedical research.
The research is set to continue throughout 2024, with the potential for further exploration into the applications of AWRP in various psychological and biomedical contexts. By bridging the gap between technology and emotional understanding, this study aims to contribute to the development of more effective emotional recognition systems.
In conclusion, Noan Yaseka’s research represents a significant step forward in the intersection of biomedical engineering and psychology. By utilizing advanced deep learning techniques and innovative methodologies, this study not only enhances our understanding of human emotions but also paves the way for future research in emotion classification and its applications in mental health and well-being.